Unlocking Business Potential with Medical Datasets for Machine Learning
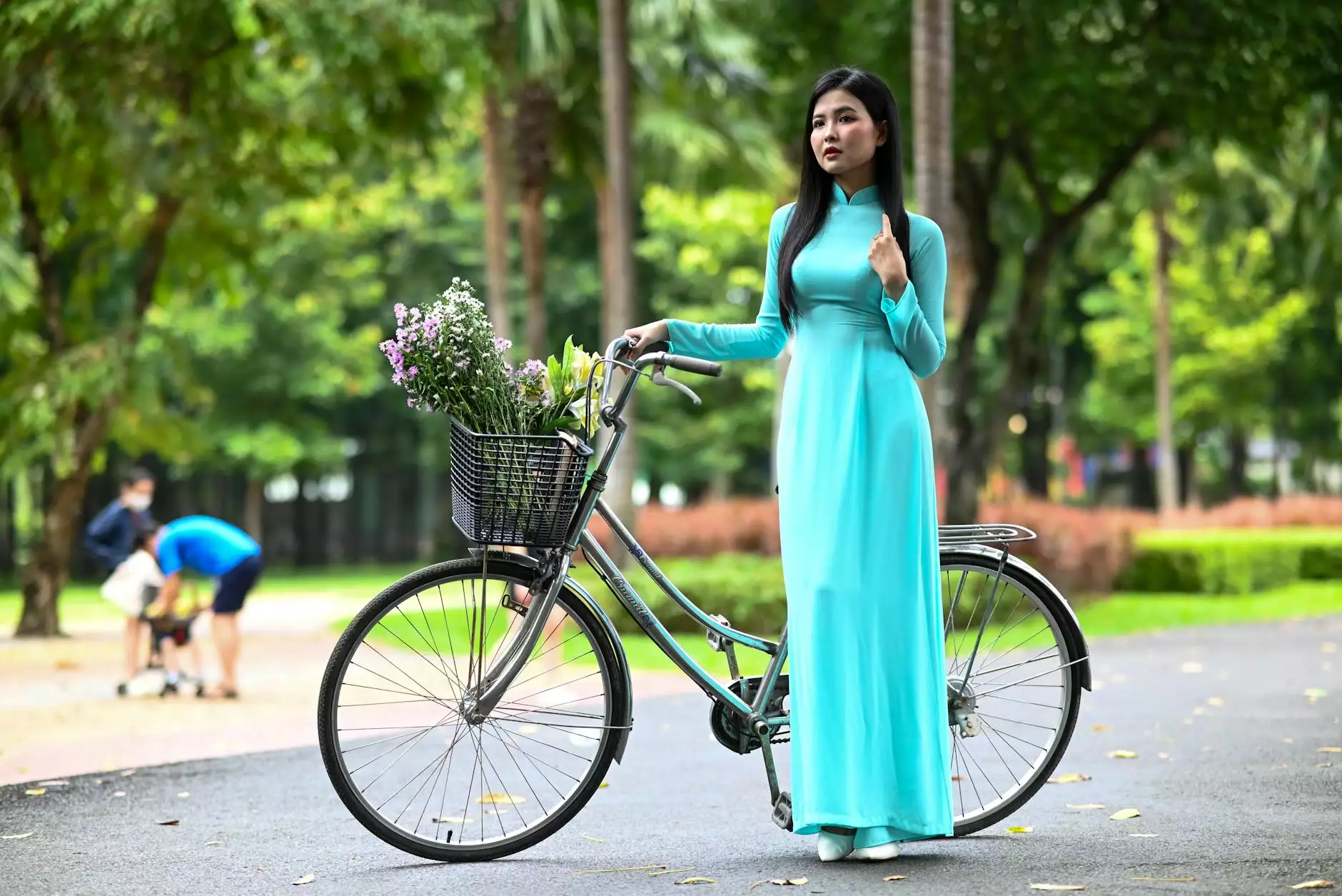
In today's rapidly evolving digital landscape, businesses are increasingly relying on data-driven insights to stay ahead of the competition. One area where this trend is particularly prominent is in the healthcare sector, where the integration of machine learning has the potential to revolutionize the industry. By utilizing a medical dataset for machine learning, organizations can gain profound insights that lead to better patient outcomes, more efficient services, and ultimately, a significant boost to their operational effectiveness.
The Importance of Medical Datasets
The backbone of any successful machine learning project is the quality of the dataset being utilized. In the case of healthcare, medical datasets include a wide range of information such as patient records, treatment outcomes, clinical trials, and even genetic data. These datasets are crucial for training machine learning models that can identify patterns, predict outcomes, and suggest the best treatment options available.
Types of Medical Datasets
There are several types of medical datasets used in machine learning:
- Electronic Health Records (EHRs): Complete patient histories including demographics, diagnoses, medications, treatment plans, and immunization dates.
- Clinical Trials Data: Information gathered from studies that test the effectiveness of new treatments.
- Genomic Data: Data derived from DNA sequencing that can help in personalized medicine.
- Medical Imaging Data: Images from X-rays, MRIs, and CT scans that can be analyzed to detect abnormalities.
How Machine Learning Transforms Healthcare
Machine learning algorithms applied to medical datasets for machine learning can lead to breakthroughs in several areas:
1. Predictive Analytics
One of the most promising applications of machine learning in healthcare is in predictive analytics. By analyzing large datasets, machine learning models can identify at-risk patients before they require acute care. This capability enables healthcare providers to take proactive measures and implement preventive strategies, significantly reducing costs and improving patient outcomes.
2. Enhanced Diagnosis
Machine learning can facilitate more accurate and faster diagnoses. For example, algorithms trained on medical imaging datasets can identify signs of diseases such as cancer at earlier stages than traditional methods. By optimizing diagnostic processes, healthcare professionals can deliver timely and effective treatment.
3. Personalized Medicine
With access to rich datasets, machine learning can drive the development of personalized medicine. This approach tailors treatment plans based on individual patient data, including genetic information, lifestyle factors, and previous responses to treatments. Such customization increases the effectiveness of treatments and minimizes adverse reactions.
Business Opportunities in Leveraging Medical Datasets
The integration of machine learning in healthcare not only improves patient care but also opens new avenues for businesses. Here are some key opportunities:
1. Development of Innovative Products
Companies utilizing medical datasets for machine learning can develop innovative healthcare products and services. For instance, AI-driven diagnostic tools and mobile health applications can enhance patient engagement and monitoring.
2. Cost Reduction and Increased Efficiency
By leveraging data analytics, businesses can significantly reduce operational costs. Automated processes powered by machine learning reduce the need for manual intervention, streamline workflows, and minimize errors, leading to heightened efficiency.
3. Competitive Advantage
Organizations that capitalize on machine learning insights from medical datasets can gain a substantial competitive edge. Early adopters of such technologies are more likely to improve their service delivery and capture larger market shares.
Challenges and Considerations
While the potential of machine learning in the healthcare sector is vast, it is essential to acknowledge the challenges involved:
1. Data Privacy and Security
One of the foremost concerns regarding the use of medical datasets is patient privacy. Compliance with regulations such as HIPAA in the United States is crucial to ensure that sensitive information is adequately protected.
2. Data Quality and Integrity
Machine learning algorithms are highly sensitive to data quality. Poorly structured or incomplete datasets can lead to inaccurate models. Therefore, investing in data cleansing and validation processes is critical.
3. Ethical Considerations
The implementation of machine learning in healthcare also raises ethical questions. For instance, how can we ensure that algorithms are unbiased and do not inadvertently amplify health disparities? Addressing these concerns is essential for building trust in AI-driven solutions.
Conclusion: Embracing Data-Driven Healthcare
As the healthcare landscape continues to transform, the integration of medical datasets for machine learning stands out as a pivotal force for change. By tapping into the vast potential of machine learning, healthcare providers and businesses alike can not only enhance their operational efficiency but also improve the quality of care delivered to patients.
The future is bright for those who are willing to embrace this data-driven approach. Investing in quality datasets, innovative technologies, and skilled expertise will define leading organizations in the healthcare sector for years to come. As we venture further into this era of transformation, the possibilities are endless for businesses that want to leverage the power of machine learning.