Leveraging Machine Learning Spam Detection in Modern Business
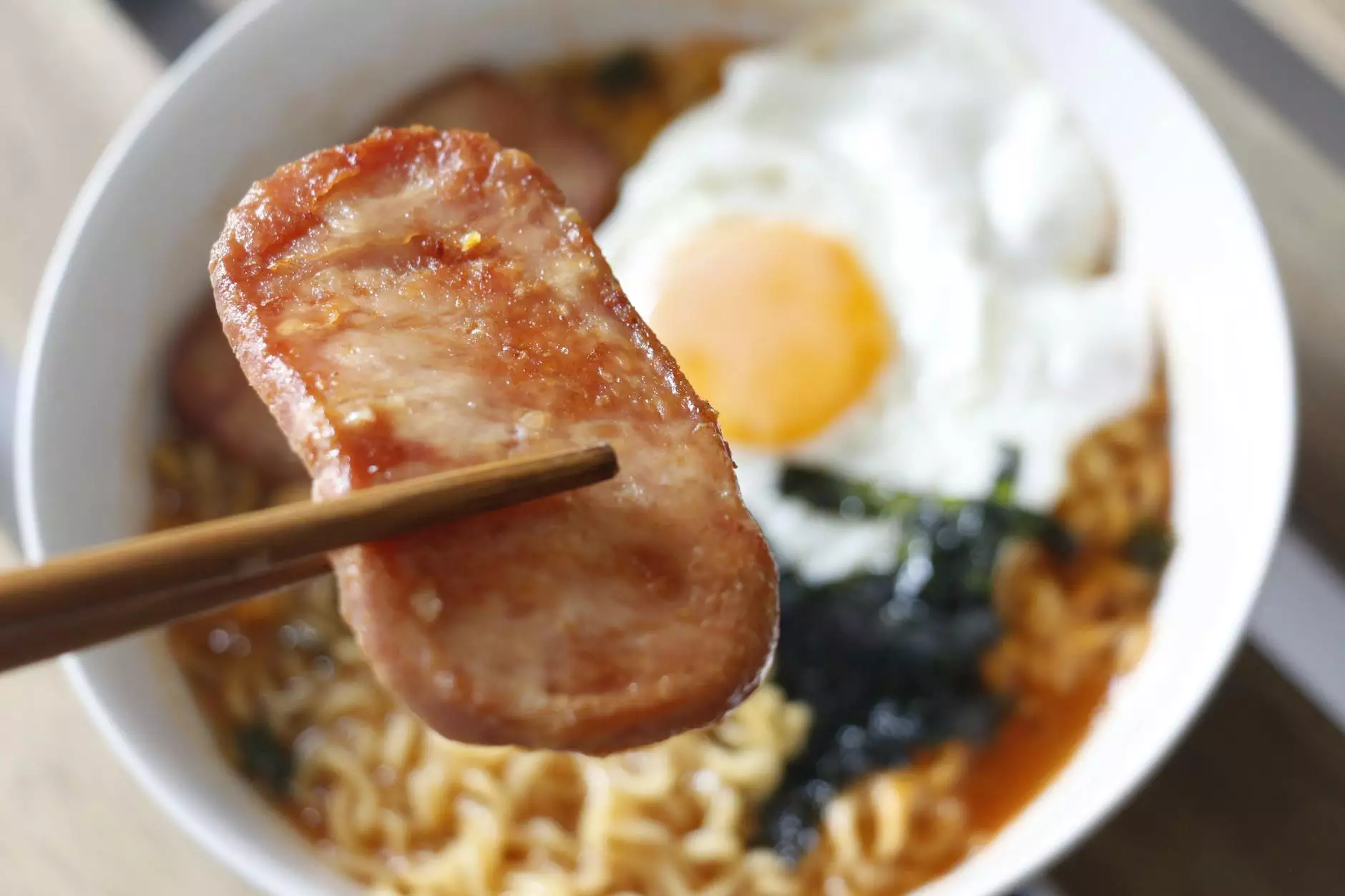
In today’s digital landscape, where businesses rely heavily on online communication and transactions, the issue of spam has become more prevalent than ever. As a result, organizations are seeking innovative solutions to combat spam effectively. One of the most powerful technologies being utilized in this domain is machine learning. This article explores the impact of machine learning on spam detection and how it can enhance IT services and security systems in businesses.
Understanding Spam and Its Implications
Spam refers to unsolicited and often irrelevant messages sent over the Internet, typically to a large number of users. These messages can take various forms, including:
- Email Spam: Unwanted advertisements or malicious links sent via email.
- Comment Spam: Irrelevant comments posted on blogs and forums.
- Social Media Spam: Promotional messages or misleading posts on social platforms.
The implications of spam are significant for businesses. In addition to being an annoyance, spam can lead to security breaches, data loss, and a tarnished brand reputation. Therefore, implementing an effective spam detection system is essential for maintaining operational integrity.
The Rise of Machine Learning in Spam Detection
Machine learning refers to the ability of software systems to improve their performance on a given task over time through experience. In the context of spam detection, machine learning algorithms can analyze vast amounts of data to identify patterns and characteristics of spam messages.
How Machine Learning Works in Spam Detection
Machine learning models utilize various techniques to filter out spam. These include:
- Data Collection: Gathering data from various sources, including email servers and user feedback.
- Feature Extraction: Identifying key features of spam, such as keywords, sender reputation, and user behavior.
- Model Training: Training the algorithm on labeled data (messages identified as spam or not spam).
- Prediction: Utilizing the trained model to classify incoming messages as spam or legitimate.
- Continuous Learning: Updating the model regularly with new data to adapt to evolving spam tactics.
The Benefits of Machine Learning in Spam Detection
Adopting machine learning for spam detection offers several advantages for businesses, including:
1. Enhanced Accuracy
Machine learning algorithms can achieve remarkable accuracy in identifying spam messages, reducing the incidence of false positives (legitimate messages marked as spam) and false negatives (spam messages that bypass filters).
2. Adaptability
Spam tactics evolve continuously. Machine learning models can be retrained on new data, allowing them to adapt to emerging spam techniques and keeping businesses one step ahead of spammers.
3. Automation
Automating spam detection frees up valuable human resources, enabling staff to focus on more strategic initiatives rather than sifting through junk mail.
4. Improved User Experience
By significantly reducing spam in users' inboxes, businesses can enhance the overall user experience, leading to higher customer satisfaction and retention rates.
Integrating Machine Learning Spam Detection with IT Services
For IT service providers like Spambrella, integrating machine learning spam detection enhances the quality of IT services offered. Here’s how:
1. Comprehensive Email Security Solutions
IT service providers can incorporate machine learning algorithms into their email security systems, providing clients with robust spam filters that are constantly learning and evolving.
2. Customizable Solutions
Machine learning allows for the development of customizable spam detection solutions tailored to specific business needs, industry regulations, and user preferences.
3. Real-time Insights
With machine learning, businesses can receive real-time insights into spam trends and emerging threats, allowing for proactive measures to be implemented quickly.
Improving Security Systems with Machine Learning
Spam is not only an inconvenience but can also pose serious security threats. Malicious spam can lead to phishing attacks, data breaches, and other cybercrimes. Here’s how machine learning enhances security systems:
1. Threat Detection
Machine learning algorithms can analyze patterns in emails and detect anomalies indicative of phishing attempts. By identifying these threats early, organizations can take swift action to protect sensitive information.
2. User Authentication
Machine learning can assist in developing sophisticated authentication systems that verify user identities based on unique usage patterns, helping to prevent unauthorized access.
3. Incident Response
In cases where spam leads to security breaches, machine learning can aid incident response teams by identifying the source and methods of the attack, speeding up the recovery process.
Challenges and Considerations
While the benefits of machine learning in spam detection are substantial, several challenges remain:
1. Data Privacy Concerns
Organizations must remain compliant with data protection regulations (e.g., GDPR) while collecting and processing data for machine learning models. Ensuring user privacy can complicate data handling practices.
2. Initial Investment
Implementing advanced machine learning spam detection systems may require significant initial investment in technology and training, which can be a barrier for smaller businesses.
3. Continuous Monitoring and Maintenance
Machine learning models must be regularly maintained and updated to ensure they function effectively. This requires ongoing resources and expertise.
The Future of Machine Learning in Spam Detection
The future of machine learning spam detection looks promising. As technology evolves, we can expect:
1. Increased Integration with Artificial Intelligence
Machine learning is just one component of AI. Future spam detection systems will leverage a broader range of AI technologies to provide even more sophisticated protection mechanisms.
2. Enhanced Predictive Capabilities
By utilizing advanced algorithms, businesses will be able to predict and prevent spam campaigns before they occur, greatly enhancing security posture.
3. Global Collaboration
As spam is a global problem, collaboration between organizations, researchers, and technology companies will be crucial in developing effective solutions that benefit all users.
Conclusion
Machine learning spam detection is revolutionizing how businesses manage email communications and security. By implementing machine learning algorithms, organizations can significantly improve their spam-filtering capabilities, enhance user satisfaction, and protect themselves against evolving cyber threats. As technology continues to advance, the integration of machine learning into IT services and security systems will become increasingly vital for organizations to stay competitive in a rapidly changing digital landscape.
With Spambrella at the forefront of IT services and security systems, businesses have access to cutting-edge solutions that leverage machine learning to mitigate spam and safeguard their operations. Embracing these technologies will not only provide immediate benefits but also pave the way for a more secure and practical approach to email and online communication in the future.